In this study, we aim to resolve the traditionally tedious and time consuming task of determining the age of abalone by constructing predictive models of the ages of abalones using other physical measures that are easier to obtain. Based on the dataset with which we are provided, we construct models of various complexities and propose the one with the highest out-of-sample prediction performance. Our model is able to control the mean squared prediction error to an infinitesimal extent, while subject to only a reasonably small model bias. In addition, we also explore the possibilities of conjecturing other interior content (in-shell information), such as shucked weights of abalone, using measurements which can be obtained prior to cracking the shell (out-of-shell information). Our results show that not only is predicting such in-shell measures with out-of-shell information feasible, but one might achieve even better results than making predictions on the ages of the abalones.
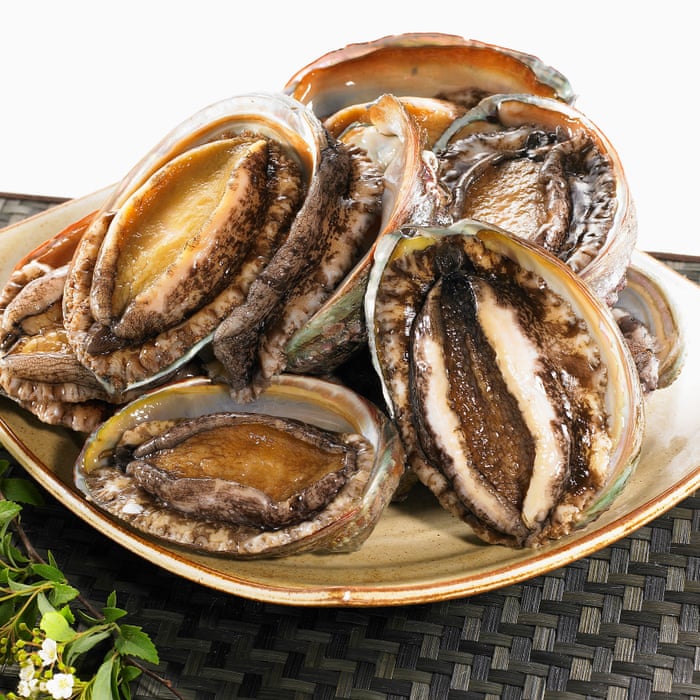